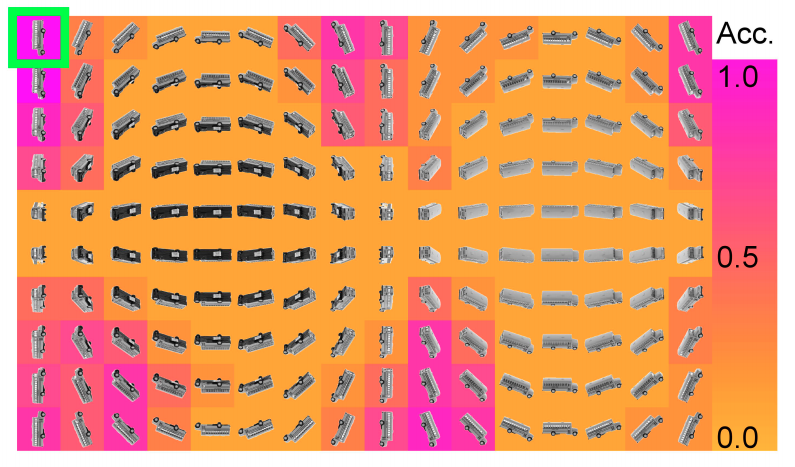
Study of the effect of viewpoint variation on performance of models trained from a single view (green highlight). Recognizing Objects From Any View With Object and Viewer-Centered Representations. Liu et al. CVPR 2020.
Research
Brief History
During my undergrad at UC San Diego I began working with Dr. Zhuowen Tu in the Machine Learning and Perception Lab (mlPC). I became quickly enamored with deep learning and continued to conduct research with the group for 2 years before beginning my masters at Georgia Tech. During my time at tech I have researched with faculty Dr. Chao Zhang on the problem of continual learning.
Publications
Liu, S., Nguyen, V., Rehg, I., & Tu, Z. (2020). Recognizing Objects From Any View With Object and Viewer-Centered Representations. 2020 IEEE/CVF Conference on Computer Vision and Pattern Recognition (CVPR), 11781-11790. [pdf]
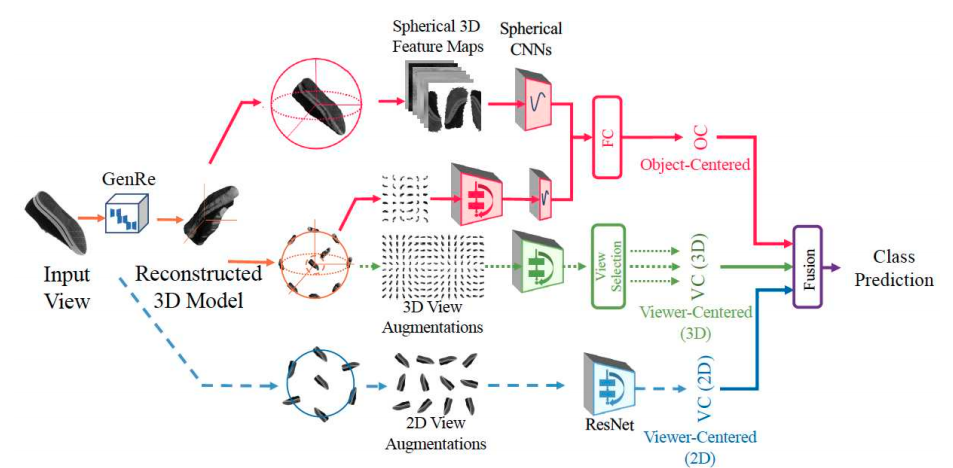
Yinghao Li, Rui Feng, Isaac Rehg, & Chao Zhang. (2020). Transformer-Based Neural Text Generation with Syntactic Guidance. [pdf]
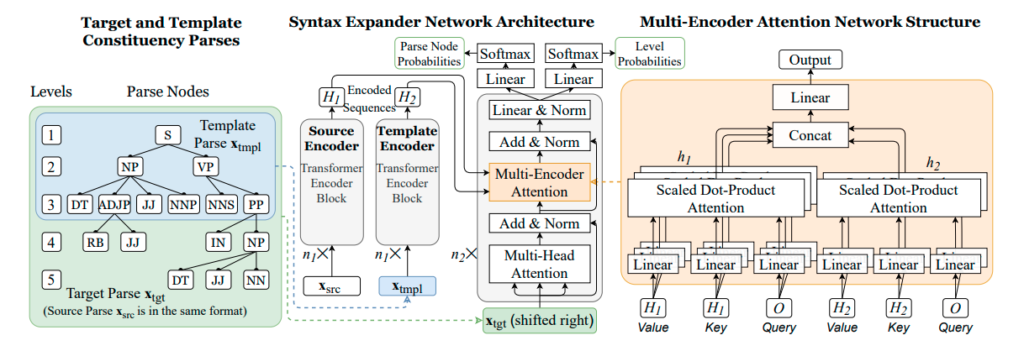
Ongoing Work
I have been collaborating with PhD candidate, Anh Thai, on research into the effect of exposure repetitions in continual learning. Our paper, Does Continual Learning = Catastrophic Forgetting?, has been submitted to CVPR 2021 and is under review.
In order to succeed in the continual learning setting, learners must decide when to consolidate information gleaned from previous tasks with that of later tasks. Under supervision of Dr. Chao Zhang, I have designed a modular architecture that enables identification and consolidation of network components with similar functionalities across separately trained models. We are targeting a submission to ICML 2021.